2. Assessment¶
2.1. Metrics¶
The fairlearn.metrics
module provides the means to assess fairness-related
metrics for models. This applies for any kind of model that users may already
use, but also for models created with mitigation techniques from the
Mitigation section. The Fairlearn dashboard provides a visual way to
compare metrics between models as well as compare metrics for different groups
on a single model.
2.1.1. Ungrouped Metrics¶
At their simplest, metrics take a set of ‘true’ values \(Y_{true}\) (from the input data) and predicted values \(Y_{pred}\) (by applying the model to the input data), and use these to compute a measure. For example, the recall or true positive rate is given by
That is, a measure of whether the model finds all the positive cases in the
input data. The scikit-learn package implements this in
sklearn.metrics.recall_score()
.
Suppose we have the following data we can see that the prediction is 1 in five of the ten cases where the true value is 1, so we expect the recall to be 0.5:
>>> import sklearn.metrics as skm
>>> y_true = [0, 1, 1, 1, 1, 0, 1, 0, 1, 0, 0, 0, 1, 1, 1, 1]
>>> y_pred = [0, 0, 1, 0, 1, 1, 1, 0, 0, 1, 1, 1, 1, 0, 0, 1]
>>> skm.recall_score(y_true, y_pred)
0.5
2.1.2. Metrics with Grouping¶
When considering fairness, each row of input data will have an associated group label \(g \in G\), and we will want to know how the metric behaves for each \(g\). To help with this, Fairlearn provides a class, which takes an existing (ungrouped) metric function, and applies it to each group within a set of data.
Suppose in addition to the \(Y_{true}\) and \(Y_{pred}\) above, we had the following set of labels:
>>> import numpy as np
>>> import pandas as pd
>>> group_membership_data = ['d', 'a', 'c', 'b', 'b', 'c', 'c', 'c',
... 'b', 'd', 'c', 'a', 'b', 'd', 'c', 'c']
>>> pd.set_option('display.max_columns', 20)
>>> pd.set_option('display.width', 80)
>>> pd.DataFrame({ 'y_true': y_true,
... 'y_pred': y_pred,
... 'group_membership_data': group_membership_data})
y_true y_pred group_membership_data
0 0 0 d
1 1 0 a
2 1 1 c
3 1 0 b
4 1 1 b
5 0 1 c
6 1 1 c
7 0 0 c
8 1 0 b
9 0 1 d
10 0 1 c
11 0 1 a
12 1 1 b
13 1 0 d
14 1 0 c
15 1 1 c
We then calculate a metric which shows the subgroups:
>>> from fairlearn.metrics import MetricFrame
>>> grouped_metric = MetricFrame(metrics=skm.recall_score,
... y_true=y_true,
... y_pred=y_pred,
... sensitive_features=group_membership_data)
>>> print("Overall recall = ", grouped_metric.overall)
Overall recall = 0.5
>>> print("recall by groups = ", grouped_metric.by_group.to_dict())
recall by groups = {'a': 0.0, 'b': 0.5, 'c': 0.75, 'd': 0.0}
Note that the overall recall is the same as that calculated above in the Ungrouped Metric section, while the ‘by group’ dictionary can be checked against the table above.
In addition to these basic scores, Fairlearn also provides convenience functions to recover the maximum and minimum values of the metric across groups and also the difference and ratio between the maximum and minimum:
>>> print("min recall over groups = ", grouped_metric.group_min())
min recall over groups = 0.0
>>> print("max recall over groups = ", grouped_metric.group_max())
max recall over groups = 0.75
>>> print("difference in recall = ", grouped_metric.difference(method='between_groups'))
difference in recall = 0.75
>>> print("ratio in recall = ", grouped_metric.ratio(method='between_groups'))
ratio in recall = 0.0
A single instance of fairlearn.metrics.MetricFrame
can evaluate multiple
metrics simultaneously (note that fairlearn.metrics.count()
can be used to
show each group’s size):
>>> from fairlearn.metrics import count
>>> multi_metric = MetricFrame({'precision':skm.precision_score,
... 'recall':skm.recall_score,
... 'count': count},
... y_true, y_pred,
... sensitive_features=group_membership_data)
>>> multi_metric.overall
precision 0.5555...
recall 0.5...
dtype: object
>>> multi_metric.by_group
precision recall count
sensitive_feature_0
a 0.0 0.0 2
b 1.0 0.5 4
c 0.6 0.75 7
d 0.0 0.0 3
If there are per-sample arguments (such as sample weights), these can also be provided
in a dictionary via the sample_params
argument.:
>>> s_w = [1, 2, 1, 3, 2, 3, 1, 2, 1, 2, 3, 1, 2, 3, 2, 3]
>>> s_p = { 'sample_weight':s_w }
>>> weighted = MetricFrame(metrics=skm.recall_score,
... y_true=y_true,
... y_pred=y_pred,
... sensitive_features=pd.Series(group_membership_data, name='SF 0'),
... sample_params=s_p)
>>> weighted.overall
0.45
>>> weighted.by_group
SF 0
a 0...
b 0.5...
c 0.7142...
d 0...
Name: recall_score, dtype: object
If multiple metrics are being evaluated, then sample_params
becomes a dictionary of
dictionaries, with the first key corresponding matching that in the dictionary holding
the desired underlying metric functions.
We do not support non-sample parameters at the current time. If these are required, then
use functools.partial()
to prebind the required arguments to the metric
function:
>>> import functools
>>> fbeta_06 = functools.partial(skm.fbeta_score, beta=0.6)
>>> metric_beta = MetricFrame(metrics=fbeta_06,
... y_true=y_true,
... y_pred=y_pred,
... sensitive_features=group_membership_data)
>>> metric_beta.overall
0.5396825396825397
>>> metric_beta.by_group
sensitive_feature_0
a 0...
b 0.7906...
c 0.6335...
d 0...
Name: metric, dtype: object
Finally, multiple sensitive features can be specified. The by_groups
property then
holds the intersections of these groups:
>>> g_2 = [ 8,6,8,8,8,8,6,6,6,8,6,6,6,6,8,6]
>>> s_f_frame = pd.DataFrame(np.stack([group_membership_data, g_2], axis=1),
... columns=['SF 0', 'SF 1'])
>>> metric_2sf = MetricFrame(metrics=skm.recall_score,
... y_true=y_true,
... y_pred=y_pred,
... sensitive_features=s_f_frame)
>>> metric_2sf.overall # Same as before
0.5
>>> metric_2sf.by_group
SF 0 SF 1
a 6 0.0
8 NaN
b 6 0.5
8 0.5
c 6 1.0
8 0.5
d 6 0.0
8 0.0
Name: recall_score, dtype: object
With such a small number of samples, we are obviously running into cases where
there are no members in a particular combination of sensitive features. In this
case we see that the subgroup (a, 8)
has a result of NaN
, indicating
that there were no samples in it.
2.1.3. Scalar Results from MetricFrame
¶
Higher level machine learning algorithms (such as hyperparameter tuners) often
make use of metric functions to guide their optimisations.
Such algorithms generally work with scalar results, so if we want the tuning
to be done on the basis of our fairness metrics, we need to perform aggregations
over the MetricFrame
.
We provide a convenience function, fairlearn.metrics.make_derived_metric()
,
to generate scalar-producing metric functions based on the aggregation methods
mentioned above (MetricFrame.group_min()
, MetricFrame.group_max()
,
MetricFrame.difference()
, and MetricFrame.ratio()
).
This takes an underlying metric function, the name of the desired transformation, and
optionally a list of parameter names which should be treated as sample aligned parameters
(such as sample_weight).
Other parameters will be passed to the underlying metric function normally (unlike
MetricFrame
where functools.partial()
must be used, as noted above).
The result is a function which builds the MetricFrame
internally and performs
the requested aggregation. For example:
>>> from fairlearn.metrics import make_derived_metric
>>> fbeta_difference = make_derived_metric(metric=skm.fbeta_score,
... transform='difference')
>>> # Don't need functools.partial for make_derived_metric
>>> fbeta_difference(y_true, y_pred, beta=0.7,
... sensitive_features=group_membership_data)
0.752525...
>>> # But as noted above, functools.partial is needed for MetricFrame
>>> fbeta_07 = functools.partial(skm.fbeta_score, beta=0.7)
>>> MetricFrame(metrics=fbeta_07,
... y_true=y_true,
... y_pred=y_pred,
... sensitive_features=group_membership_data).difference()
0.752525...
We use fairlearn.metrics.make_derived_metric()
to manufacture a number
of such functions which will be commonly used:
Base metric |
|
|
|
|
---|---|---|---|---|
. |
. |
Y |
Y |
|
. |
. |
Y |
Y |
|
. |
. |
Y |
Y |
|
. |
. |
Y |
Y |
|
. |
. |
Y |
Y |
|
Y |
. |
Y |
Y |
|
Y |
. |
. |
. |
|
Y |
. |
. |
. |
|
. |
Y |
. |
. |
|
. |
Y |
. |
. |
|
. |
Y |
. |
. |
|
Y |
. |
. |
. |
|
Y |
. |
. |
. |
|
Y |
. |
. |
. |
|
Y |
. |
. |
. |
|
. |
Y |
Y |
Y |
The names of the generated functions are of the form
fairlearn.metrics.<base_metric>_<transformation>
.
For example fairlearn.metrics.accuracy_score_difference
and
fairlearn.metrics.precision_score_group_min
.
2.1.4. Control features for grouped metrics¶
Control features (sometimes called ‘conditional’ features) enable more detailed
fairness insights by providing a further means of splitting the data into
subgroups.
When the data are split into subgroups, control features (if provided) act
similarly to sensitive features.
However, the ‘overall’ value for the metric is now computed for each subgroup
of the control feature(s).
Similarly, the aggregation functions (such as MetricFrame.group_max
) are
performed for each subgroup in the conditional feature(s), rather than across
them (as happens with the sensitive features).
Control features are useful for cases where there is some expected variation with a feature, so we need to compute disparities while controlling for that feature. For example, in a loan scenario we would expect people of differing incomes to be approved at different rates, but within each income band we would still want to measure disparities between different sensitive features. However, it should be borne in mind that due to historic discrimination, the income band might be correlated with various sensitive features. Because of this, control features should be used with particular caution.
The MetricFrame
constructor allows us to specify control features in
a manner similar to sensitive features, using a conditional_features=
parameter:
>>> decision = [
... 0,0,0,1,1,0,1,1,0,1,
... 0,1,0,1,0,1,0,1,0,1,
... 0,1,1,0,1,1,1,1,1,0
... ]
>>> prediction = [
... 1,1,0,1,1,0,1,0,1,0,
... 1,0,1,0,1,1,1,0,0,0,
... 1,1,1,0,0,1,1,0,0,1
... ]
>>> control_feature = [
... 'H','L','H','L','H','L','L','H','H','L',
... 'L','H','H','L','L','H','L','L','H','H',
... 'L','H','L','L','H','H','L','L','H','L'
... ]
>>> sensitive_feature = [
... 'A','B','B','C','C','B','A','A','B','A',
... 'C','B','C','A','C','C','B','B','C','A',
... 'B','B','C','A','B','A','B','B','A','A'
... ]
>>> metric_c_f = MetricFrame(metrics=skm.accuracy_score,
... y_true=decision,
... y_pred=prediction,
... sensitive_features={'SF' : sensitive_feature},
... control_features={'CF' : control_feature})
>>> # The 'overall' property is now split based on the control feature
>>> metric_c_f.overall
CF
H 0.4285...
L 0.375...
Name: accuracy_score, dtype: object
>>> # The 'by_group' property looks similar to how it would if we had two sensitive features
>>> metric_c_f.by_group
CF SF
H A 0.2...
B 0.4...
C 0.75...
L A 0.4...
B 0.2857...
C 0.5...
Name: accuracy_score, dtype: object
Note how the MetricFrame.overall
property is stratified based on the
supplied control feature. The MetricFrame.by_group
property allows
us to see disparities between the groups in the sensitive feature for each
group in the control feature.
When displayed like this, MetricFrame.by_group
looks similar to
how it would if we had specified two sensitive features (although the
control features will always be at the top level of the hierarchy).
With the MetricFrame
computed, we can perform aggregations:
>>> # See the maximum accuracy for each value of the control feature
>>> metric_c_f.group_max()
CF
H 0.75
L 0.50
Name: accuracy_score, dtype: float64
>>> # See the maximum difference in accuracy for each value of the control feature
>>> metric_c_f.difference(method='between_groups')
CF
H 0.55...
L 0.2142...
Name: accuracy_score, dtype: float64
In each case, rather than a single scalar, we receive one result for each
subgroup identified by the conditional feature. The call
metric_c_f.group_max()
call shows the maximum value of the metric across
the subgroups of the sensitive feature within each value of the control feature.
Similarly, metric_c_f.difference(method='between_groups')
call shows the
maximum difference between the subgroups of the sensitive feature within
each value of the control feature.
For more examples, please
see the Metrics with Multiple Features notebook in the
Example Notebooks.
2.1.5. Plotting grouped metrics¶
The simplest way to visualize grouped metrics from the MetricFrame
is
to take advantage of the inherent plotting capabilities of
pandas.DataFrame
:
metrics = {
'accuracy': accuracy_score,
'precision': precision_score,
'recall': recall_score,
'false positive rate': false_positive_rate,
'true positive rate': true_positive_rate,
'selection rate': selection_rate,
'count': count}
metric_frame = MetricFrame(metrics=metrics,
y_true=y_true,
y_pred=y_pred,
sensitive_features=sex)
metric_frame.by_group.plot.bar(
subplots=True,
layout=[3, 3],
legend=False,
figsize=[12, 8],
title="Show all metrics",
)
It is possible to customize the plots. Here are some common examples.
2.1.5.1. Customize Plots: ylim
¶
The y-axis range is automatically set, which can be misleading, therefore it is sometimes useful to set the ylim argument to define the yaxis range.
metric_frame.by_group.plot(
kind="bar",
ylim=[0, 1],
subplots=True,
layout=[3, 3],
legend=False,
figsize=[12, 8],
title="Show all metrics with assigned y-axis range",
)
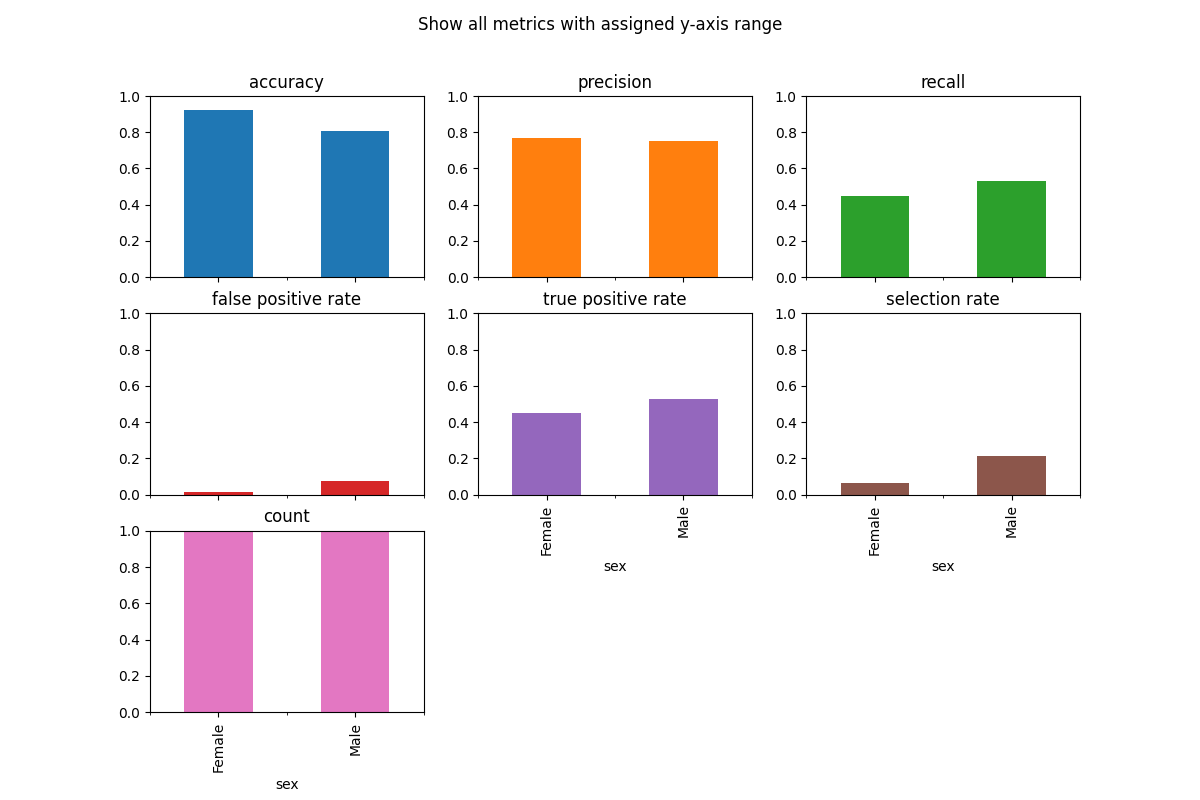
2.1.5.2. Customize Plots: colormap
¶
To change the color scheme, we can use the colormap argument. A list of colorschemes can be found here.
metric_frame.by_group.plot(
kind="bar",
subplots=True,
layout=[3, 3],
legend=False,
figsize=[12, 8],
colormap="Accent",
title="Show all metrics in Accent colormap",
)
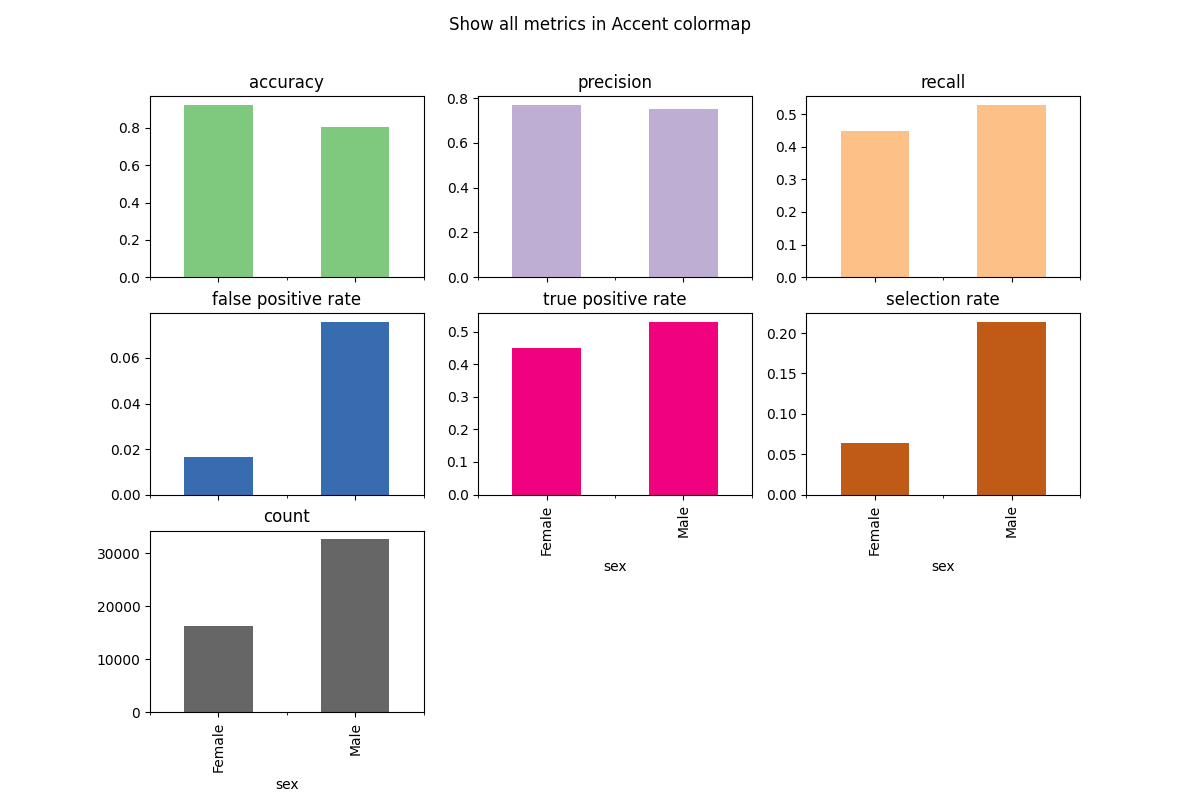
2.1.5.3. Customize Plots: kind
¶
There are different types of charts (e.g. pie, bar, line) which can be defined by the kind argument. Here is an example of a pie chart.
metric_frame.by_group.plot(
kind="pie",
subplots=True,
layout=[3, 3],
legend=False,
figsize=[12, 8],
title="Show all metrics in pie",
)
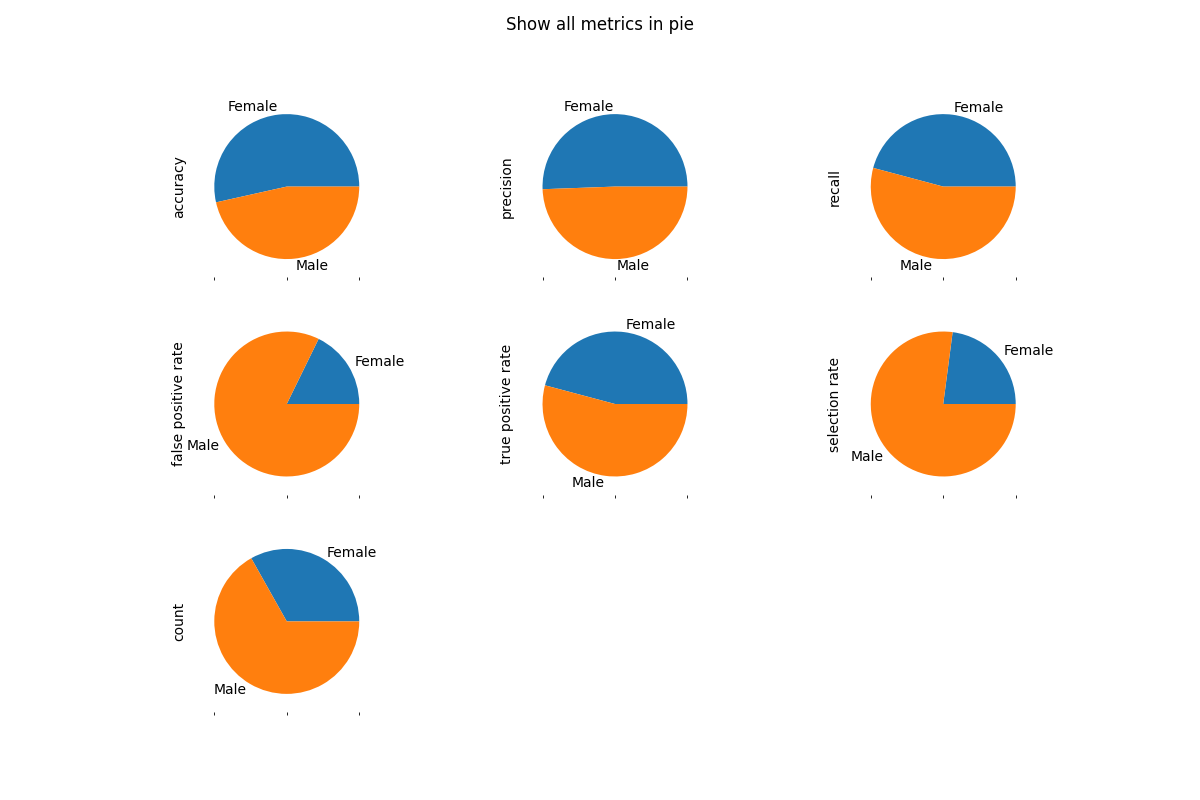
There are many other customizations that can be done. More information can be found in
pandas.DataFrame.plot()
.
2.2. Fairlearn dashboard¶
The Fairlearn dashboard was a Jupyter notebook widget for assessing how a model’s predictions impact different groups (e.g., different ethnicities), and also for comparing multiple models along different fairness and performance metrics.
Note
The FairlearnDashboard
is no longer being developed as
part of Fairlearn.
For more information on how to use it refer to
https://github.com/microsoft/responsible-ai-widgets.
Fairlearn provides some of the existing functionality through
matplotlib
-based visualizations. Refer to the Plotting grouped metrics section.